Dear Colleagues,I am pleased to let you know that I have been appointed as Editorial Board of ComTech and kindly invite you to submit your works to journal ComTech. ComTech is a semi-annual journal, published the issue every June and December. The journal invites professionals in education, research, and entrepreneurship to participate in disseminating ideas, concepts, new theories, or science development in the field of Information Systems, Architecture, Civil Engineering, Computer Engineering, Industrial Engineering, Food Technology, Computer Science, Mathematics, and Statistics. ComTech has been accredited by Directorate General of Higher Education, Ministry of Education and Culture, Republic of Indonesia (DIKTI) under the decree number 3/E/KPT/2019 (SINTA 2) and indexed by CrossRef, ASEAN Citation Index, Directory of Open Access Journals (DOAJ), Science and Technology Index 2 (SINTA2), Garda Rujukan Digital (Garuda), Microsoft Academic Search, Google Scholar, etc. There will be an article-processing charge (APC) for the accepted papers to publish the paper under Open Access license (Creative Commons Attribution-ShareAlike 4.0 International License). The APC fee is Rp. 2.000.000,00 (IDR) and the author will receive a complimentary hard copy of our journal. However, the APC fee is
FREE-of-CHARGE for international authors.
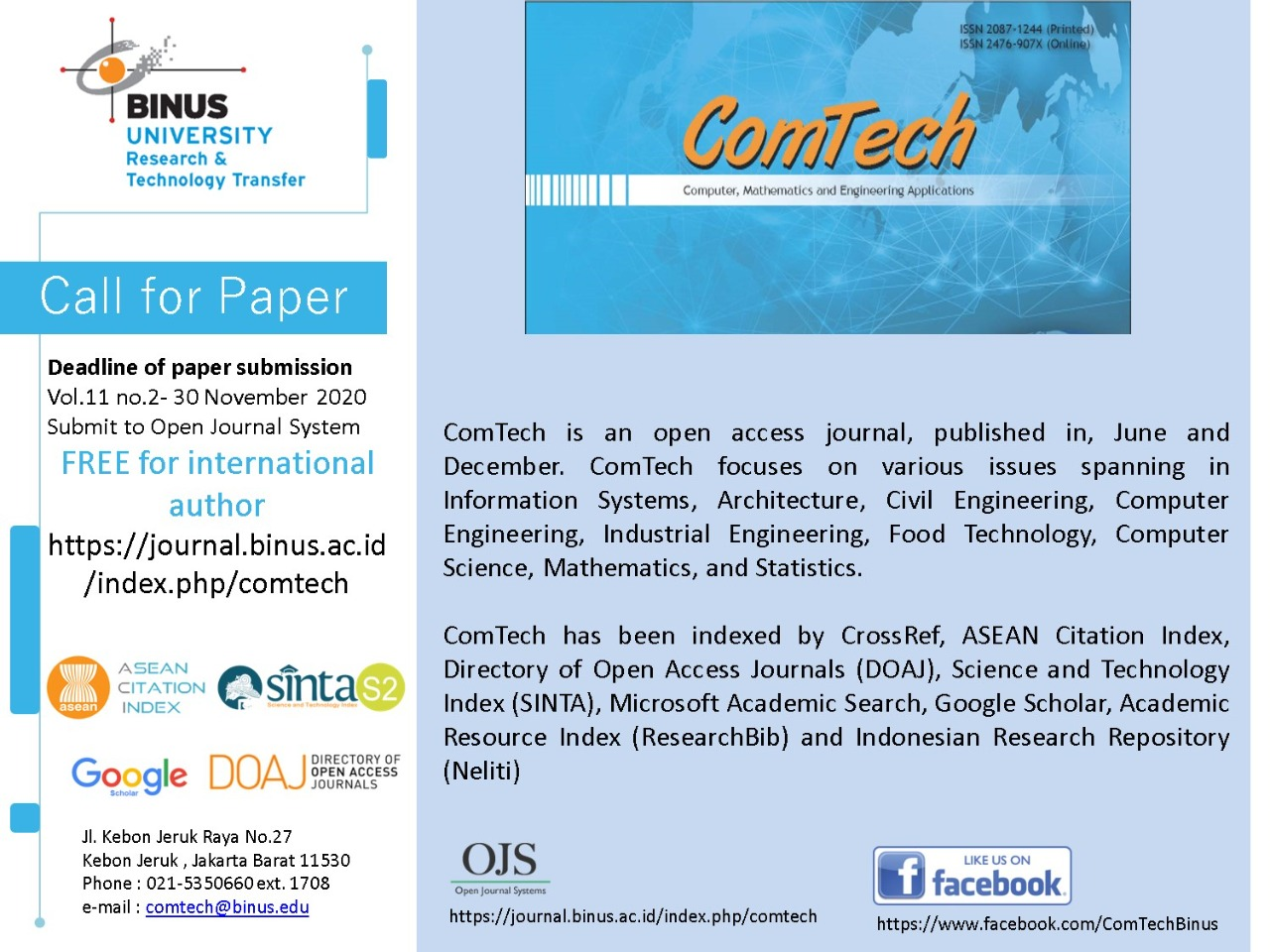
Please consider contributing to this journal and thank you for your consideration.
Dr. Muhammad Syafrudin
Editorial BoardJournal link:
ComTech