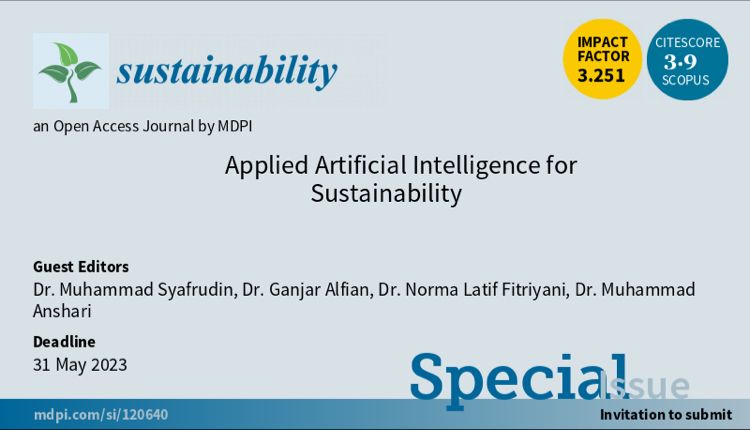
My colleagues and I are guest editing a special issue “Applied Artificial Intelligence for Sustainability”, which will be published continuously by Sustainability (SSCI SCIE JCR Q2, Scopus SJR Q1).
My colleagues and I are guest editing a special issue “Applied Artificial Intelligence for Sustainability”, which will be published continuously by Sustainability (SSCI SCIE JCR Q2, Scopus SJR Q1).
Abstract
As the risk of diseases diabetes and hypertension increases, machine learning algorithms are being utilized to improve early stage diagnosis. This study proposes a Hybrid Prediction Model (HPM), which can provide early prediction of type 2 diabetes (T2D) and hypertension based on input risk-factors from individuals. The proposed HPM consists of Density-based Spatial Clustering of Applications with Noise (DBSCAN)-based outlier detection to remove the outlier data, Synthetic Minority Over-Sampling Technique (SMOTE) to balance the distribution of class, and Random Forest (RF) to classify the diseases. Three benchmark datasets were utilized to predict the risk of diabetes and hypertension at the initial stage. The result showed that by integrating DBSCAN-based outlier detection, SMOTE, and RF, diabetes and hypertension could be successfully predicted. The proposed HPM provided the best performance result as compared to other models for predicting diabetes as well as hypertension. Furthermore, our study has demonstrated that the proposed HPM can be applied in real cases in the IoT-based Health-care Monitoring System, so that the input risk-factors from end-user android application can be stored and analyzed in a secure remote server. The prediction result from the proposed HPM can be accessed by users through an Android application; thus, it is expected to provide an effective way to find the risk of diabetes and hypertension at the initial stage.
Published in: Applied Sciences
DOI: 10.3390/app8081325
Abstract
Current technology provides an efficient way of monitoring the personal health of individuals. Bluetooth Low Energy (BLE)-based sensors can be considered as a solution for monitoring personal vital signs data. In this study, we propose a personalized healthcare monitoring system by utilizing a BLE-based sensor device, real-time data processing, and machine learning-based algorithms to help diabetic patients to better self-manage their chronic condition. BLEs were used to gather users’ vital signs data such as blood pressure, heart rate, weight, and blood glucose (BG) from sensor nodes to smartphones, while real-time data processing was utilized to manage the large amount of continuously generated sensor data. The proposed real-time data processing utilized Apache Kafka as a streaming platform and MongoDB to store the sensor data from the patient. The results show that commercial versions of the BLE-based sensors and the proposed real-time data processing are sufficiently efficient to monitor the vital signs data of diabetic patients. Furthermore, machine learning–based classification methods were tested on a diabetes dataset and showed that a Multilayer Perceptron can provide early prediction of diabetes given the user’s sensor data as input. The results also reveal that Long Short-Term Memory can accurately predict the future BG level based on the current sensor data. In addition, the proposed diabetes classification and BG prediction could be combined with personalized diet and physical activity suggestions in order to improve the health quality of patients and to avoid critical conditions in the future.
Published in: Sensors
DOI: 10.3390/s18072183